Unveiling Credit Modeling: Unlocking Financial Forecasting
If there's one thing that has always puzzled me about the world of finance, it's the seemingly mystical ability of banks and lenders to determine our creditworthiness with just a few strokes on their keyboards. How do they make those predictions? What underlying factors do they consider? Unveiling credit modeling, the process behind financial forecasting, holds the key to answering these questions and demystifying the art of evaluating credit.
So buckle up as we embark on a fascinating journey into the intricate world of credit modeling, where math, data, and human behavior converge to unveil the secrets of financial forecasting.
Understanding Credit Modeling
What is Credit Modeling?
Credit modeling is the process of using mathematical algorithms and statistical techniques to analyze credit data and predict future credit behavior. It involves collecting relevant data, assessing risk factors, and developing models to forecast creditworthiness. By considering factors such as payment history, income, and debt levels, credit modeling helps financial institutions determine the likelihood of an individual or company defaulting on loans or credit obligations.
These models are alsoused to assess the risk associated with lending to different borrowers and to make informed decisions about interest rates and credit limits. Credit modeling enables lenders to better evaluate creditworthiness, minimize risks, and make more accurate financial forecasts.
Importance of Credit Modeling
Credit modeling is of utmost importance in the financial industry as it enables institutions to assess credit risk accurately. By leveraging historical data and statistical methods, credit modeling provides insights into the potential default or delinquency of borrowers. This information aids financial institutions in making informed decisions regarding lending and investment opportunities.
For example, credit modeling helps institutions determine interest rates, loan approvals, and credit limits.
Additionally, it assists in mitigating risk by identifying high-risk borrowers and implementing appropriate risk management strategies. By incorporating and refining credit modeling techniques, financial institutions can improve their overall risk assessment and make better-informed decisions.
Real-Life Examples of Credit Modeling
- Banks and financial institutions rely heavily on credit modeling to assess borrowers' creditworthiness and make informed lending decisions.
- Credit modeling helps lenders evaluate the risk associated with lending to an individual or a company by analyzing various factors such as credit history, income, debt-to-income ratio, and industry trends.
- For example, lenders may use credit scores derived from credit models to determine interest rates, set credit limits, or approve loan applications.
- Credit modeling also plays a significant role in risk management by identifying potential defaulters and predicting loan delinquencies.
- Through credit modeling, financial institutions can effectively manage loan portfolios, minimize losses, and maintain a healthy credit ecosystem.
Example 1: Credit Modeling at JPMorgan Chase
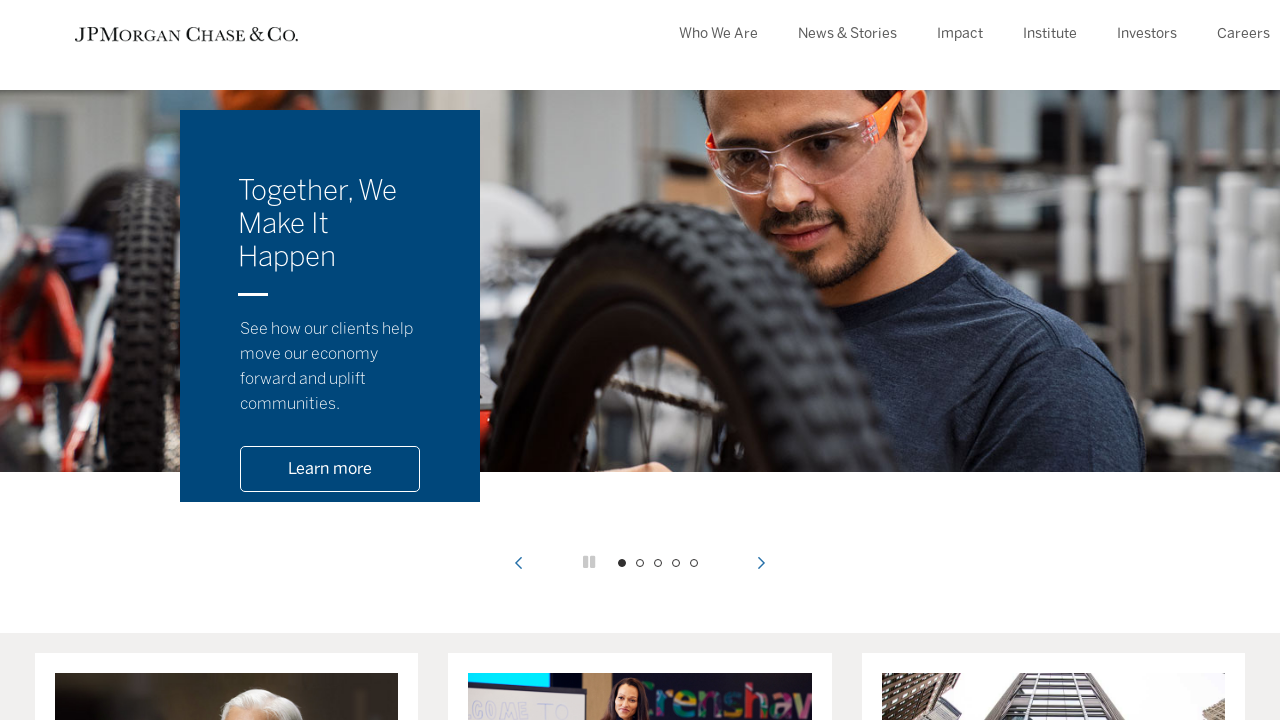
JPMorgan Chase utilizes credit modeling extensively to assess the creditworthiness of borrowers. By analyzing a wide range of data points, such as credit history, income, and financial ratios, JPMorgan Chase is able to accurately predict the likelihood of default and manage risk effectively. This information helps them make informed lending decisions and set interest rates that reflect the level of risk associated with each borrower.
Credit modeling at JPMorgan Chase also allows them to identify trends and patterns in borrower behavior, enabling them to refine their lending criteria and improve overall portfolio performance.
Example 2: Credit Modeling at Goldman Sachs
Goldman Sachs, a leading financial institution, utilizes credit modeling to assess borrower creditworthiness and manage risk. By analyzing historical data, economic indicators, and credit risk factors, Goldman Sachs develops sophisticated credit models to evaluate the likelihood of default and calculate appropriate interest rates. These models consider a range of variables, such as income, debt levels, and repayment history, to generate comprehensive credit risk profiles.
This enables the institution to make informed lending decisions, determine suitable credit limits, and set appropriate pricing. Goldman Sachs' credit modeling approach allows them to mitigate risks and ensure the financial stability of their portfolio while providing optimal lending solutions to borrowers.
Key Components of Credit Modeling
Data Collection and Analysis
Accurate and comprehensive data collection is fundamental in credit modeling. Gathering relevant financial information such as credit history, income details, and payment patterns enables the creation of robust models. Various datasets are analyzed to understand trends and patterns that affect creditworthiness. For instance, factors like debt-to-income ratio, employment history, and industry-specific data can provide insights on borrower risk profiles. Utilizing sophisticated data analysis techniques like statistical modeling and machine learning helps in developing predictive credit models. By continuously updating and refining these models with new data, lenders can make more informed decisions regarding loan approvals, interest rates, and credit limits.
Risk Assessment and Scoring
Risk assessment and scoring is a core component of credit modeling, providing valuable insights into the creditworthiness of borrowers. It involves analyzing various factors like past financial behavior, credit history, income stability, and industry performance to evaluate the level of risk associated with a loan or credit application.
By assigning a numerical score to each borrower, lenders are able to make informed decisions regarding the likelihood of repayment and the appropriate interest rates to set. For instance, a borrower with a high credit score and a consistent repayment history is considered less risky compared to someone with a low score and a history of defaults. This information helps lenders manage risk effectively and offer tailored credit options to different customer segments.
Developing Credit Models
Developing credit models is a dynamic process that involves analyzing historical data, identifying relevant variables, and creating mathematical models to predict creditworthiness. Models are built using statistical techniques like regression analysis and machine learning algorithms. It's important to strike a balance between model complexity and interpretability to ensure practical usability.
A well-developed credit model takes into account factors such as payment history, debt levels, and income stability. Regular model updates and recalibrations are necessary to adapt to changing economic conditions and maintain accuracy. By continuously refining and testing credit models, financial institutions can make informed lending decisions and mitigate risks effectively.
Benefits of Credit Modeling
Improved Accuracy in Financial Forecasting
Improved accuracy in financial forecasting is a significant advantage of credit modeling. By leveraging historical data and analyzing various credit factors, credit models enhance the precision of predicting future financial outcomes. This enables lenders and financial institutions to make more informed decisions about creditworthiness, loan approvals, and risk management.
For example, credit models can assess the likelihood of default or delinquency by analyzing variables such as payment history, income stability, and debt-to-income ratio. By incorporating these predictive insights, lenders can better anticipate potential risks and tailor their lending strategies accordingly, leading to more accurate financial forecasting and improved overall decision-making processes.
Better Risk Management and Decision Making
- Credit modeling plays a vital role in improving risk management and decision-making processes for financial institutions.
- By utilizing credit models, organizations can assess the creditworthiness of borrowers and evaluate the potential risks associated with lending to them.
- These models help identify high-risk borrowers and enable businesses to make informed decisions regarding loan approvals, interest rates, and credit limits.
- Through accurate risk assessment, institutions can mitigate the risk of defaults and minimize financial losses.
- Using credit modeling techniques, financial institutions can also optimize their portfolio allocation strategies and allocate resources more efficiently.
- By incorporating historical data, economic indicators, and predictive analytics, credit models provide valuable insights for risk assessment and enable better decision making for lenders.
Enhanced Understanding of Creditworthiness
Enhanced understanding of creditworthiness is a significant outcome of credit modeling. By analyzing diverse data points such as financial history, payment patterns, and credit utilization, credit models provide a comprehensive assessment of an individual or company's creditworthiness. This enables lenders and financial institutions to make informed decisions about granting loans or extending credit.
Challenges and Limitations of Credit Modeling
Data Availability and Quality
Data availability and quality are fundamental aspects of credit modeling. Insufficient or unreliable data can significantly impact the accuracy and effectiveness of credit models. For instance, incomplete financial records may lead to incomplete assessments of an individual's creditworthiness. Similarly, outdated or inaccurate information can misrepresent a borrower's financial health and risk profile.
To address this, organizations should ensure robust data collection processes and implement quality control measures. This involves regularly updating and validating data sources, leveraging multiple data points, and utilizing data enrichment techniques. By prioritizing data availability and quality, credit models can generate more reliable predictions and enable informed decision-making in lending and risk management.
Model Complexity and Interpretability
Credit modeling involves the development of intricate models to assess credit risk. However, model complexity can pose challenges in terms of interpretability. In practice, it is crucial to strike a balance between model sophistication and explainability. Complex models may provide more accurate predictions, but they can be difficult to understand and trust. On the other hand, overly simplistic models may lack precision. Finding the right level of complexity ensures that the credit model is both practical and insightful. For instance, using interpretable features like credit history and income level can contribute to a more transparent credit modeling process, enabling lenders to make informed decisions.
Changing Economic Conditions
In credit modeling, considering changing economic conditions is vital for accurate forecasting. Economic factors such as interest rates, inflation, and unemployment rates can significantly impact creditworthiness. For instance, during a recession, default rates tend to increase, affecting risk assessments. To account for these fluctuations, credit models should incorporate dynamic variables that adapt to economic changes. This ensures that the creditworthiness of individuals or businesses is accurately assessed based on the prevailing economic landscape. By incorporating changing economic conditions into credit modeling, lenders can make better-informed decisions and mitigate potential risks associated with economic downturns.
Future Trends in Credit Modeling
Incorporation of Machine Learning and AI
Incorporating machine learning and AI into credit modeling has revolutionized the accuracy and efficiency of financial forecasting. These advanced technologies enable the analysis of vast amounts of data, uncovering hidden patterns and risk indicators that traditional methods may overlook. Machine learning algorithms can learn from historical credit data to develop predictive models that can assess creditworthiness with greater precision.
For example, these models can identify correlations between online shopping behavior and default risk.
The use of machine learning and AI in credit modeling allows for more dynamic and adaptive models that can continuously learn and improve. By leveraging these technologies, financial institutions can make better-informed decisions, mitigate risks, and optimize lending strategies. It is crucial for organizations to embrace and invest in these advancements to stay competitive and ensure accurate credit assessments in an ever-evolving financial landscape.
Integration of Alternative Data Sources
In credit modeling, integrating alternative data sources has become increasingly valuable. These non-traditional sources provide additional insights beyond traditional financial data, allowing for a more comprehensive assessment of creditworthiness.
For example, analyzing social media activity, online purchasing behavior, or utility bill payments can offer valuable indicators of an individual's financial habits and reliability. By incorporating these alternative data sources, credit models can generate more accurate predictions and enhance risk assessment. This approach enables lenders to make more informed decisions, particularly for individuals without a long credit history. It also promotes financial inclusion by expanding access to credit for previously underserved populations.
Focus on Explainable and Fair Models
To ensure fairness and transparency, credit modeling is increasingly focusing on the development of explainable and fair models. These models aim to provide clear insights into the factors that influence credit decisions, allowing stakeholders to understand the reasoning behind the model's output. By using interpretable methodologies, such as decision trees or rule-based systems, institutions can explain how creditworthiness is assessed more effectively.
Additionally, incorporating fairness metrics helps identify and mitigate biases that may disproportionately affect certain groups. This approach promotes trust in the credit modeling process among regulators, borrowers, and other stakeholders, fostering a more inclusive and equitable financial ecosystem.
Summary
In the article "Unveiling Credit Modeling: Unlocking Financial Forecasting," the author explores the importance of credit modeling in predicting financial outcomes. Credit models are statistical tools that help institutions assess the creditworthiness of individuals and businesses. By analyzing various factors such as payment history, income, and debt levels, credit models provide insights into the likelihood of borrowers defaulting on their loans.
This information is crucial for financial institutions to make informed decisions on lending, risk management, and profitability. Through credit modeling, lenders can minimize potential losses and optimize their lending strategies. In today's data-driven world, accurate credit modeling plays a vital role in unlocking the potential of financial forecasting and improving overall credit risk management.